Capacity Accelerator Network (CAN)
The Capacity Accelerator Network is building a workforce of purpose-driven data practitioners worldwide and enabling social impact organizations to unlock the power of data to meet their missions.
Overview
data.org is committed to training one-million purpose-driven data practitioners across the globe by 2032. To achieve this ambitious goal, CAN is empowering a more diverse pool of data for social impact talent, inclusive of historically marginalized people and communities, and trained with interdisciplinary skills to combat our greatest social and environmental challenges. With these skills, this workforce will create and engage with digital public infrastructure to transform the ecosystem.
Training one-million data practitioners in the next decade is a goal that cannot be achieved alone. Collaboration and the power of partnerships are at the heart of data.org’s focus on capacity development. With accelerators based in the US, India, and across Africa, CAN is driving data for social impact in financial inclusion and the intersection of climate and health through training, curriculum development, and experiential learning opportunities with a key focus on inclusion, diversity, equity, and accessibility (IDEA).
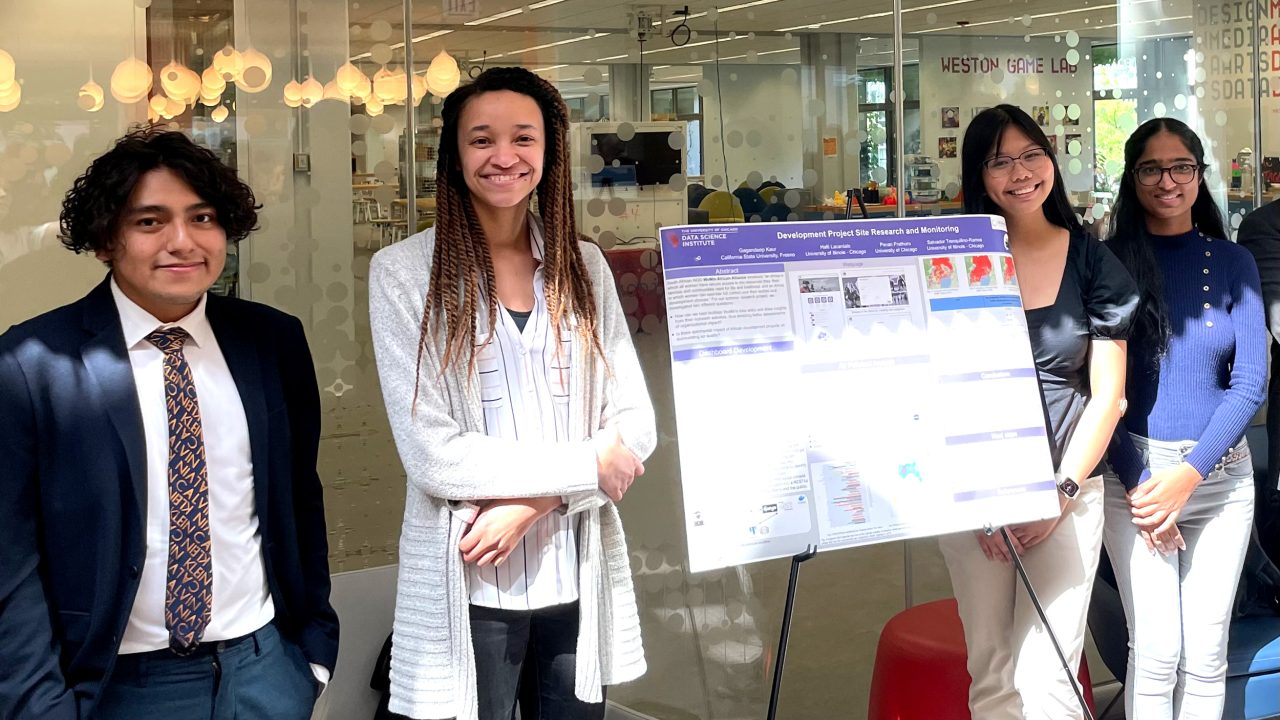
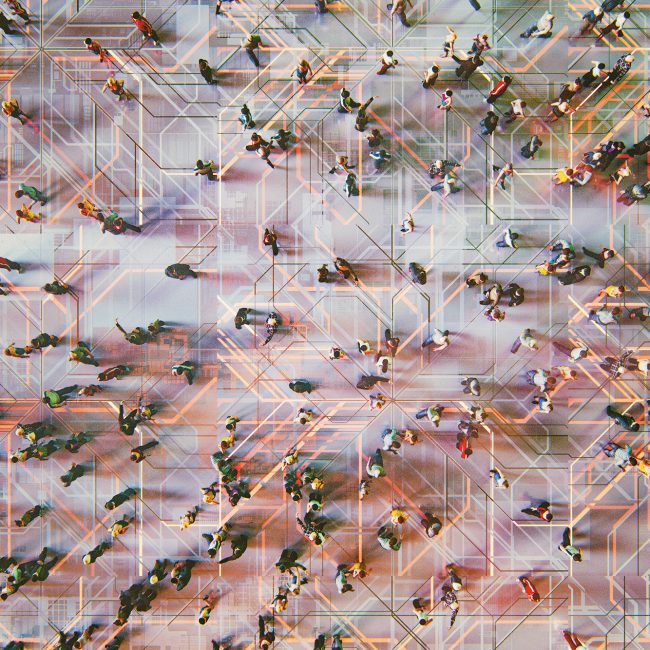
Global DSI Network
The Global DSI Network is a community of practice where members can share resources, offer training, and aggregate market insights. This draft charter is open for review and comment.
Data Training in Action
Explore the global network of local partners and learn about their work developing data for social impact talent, curricula, and resources.
-
United States of America
data.org’s US Financial Inclusion Accelerator focuses on advancing data for social impact curricula, with an emphasis on financial inclusion to address systemic issues of inequity. data.org partnered with UChicago to identify seven additional partners to drive this work forward.
-
Africa
In partnership with The Global Partnership for Sustainable Development Data (GPSDD) and their lead training partner, The African Population and Health Research Center (APHRC), the Africa Accelerator is providing social impact and government professional with interdisciplinary subject-matter expertise in climate and health to accelerating career pathways and contribute to the growth of the social sector.
-
India
In partnership with J-PAL South Asia and three leading university partners, the India Accelerator will produce a cohort of purpose-driven data practitioners with interdisciplinary subject-matter expertise in climate and health data with a key focus on inclusion, diversity, equity, and accessibility (IDEA) and intersectionality to effectively drive social impact.
Against a backdrop of the climate crisis, pandemics, and rising inequality, the world faces enormous, systemic challenges. Data science and other data-driven technologies can offer part of the solution through their tremendous ability to scale, but only if we ensure these data professionals are equipped with the interdisciplinary skills to ask the right questions and build trust with the communities they serve.
Danil Mikhailov, Ph.D. Executive Director data.org
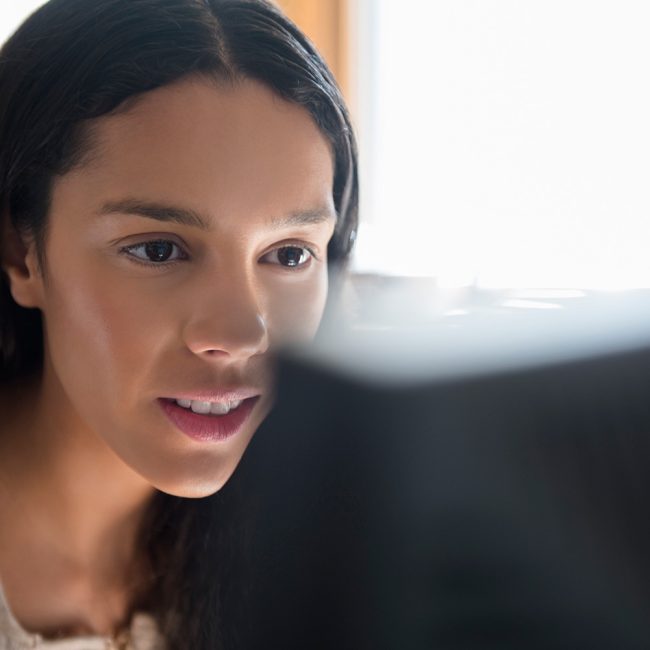
Report
Workforce Wanted: Data Talent for Social Impact
Workforce Wanted: Data Talent for Social Impact is a first-of-its-kind report on global data talent in the social sector. Confronting systemic challenges and highlighting both immediate and big-picture opportunities, this report delivers the current landscape and reveals four pathways forward for building purpose-driven data professionals.
Building the Field of Data for Social Impact
Learn more about data.org’s field-building mission and the critical need to develop purpose-driven data capacity across the globe.
Related Articles & Impact Stories
Latest updates and thought leadership posts about the Capacity Accelerator Network (CAN) initiative.