After a two-year COVID hiatus, conferences are back! I spoke at three in-person conferences in quick succession over the past three weeks: CogX in London, SciFoo in Silicon Valley, and the Aspen Ideas Festival. For those not familiar with them, the first is a premier tech and innovation event, the second focuses on cutting-edge science and technology and the third engages with ideas in art, tech, and business.
These events are certainly far from being representative, either in their focus or in their invitee list so we should be cautious about drawing any broad conclusions about what is important in the world at large. However, one area the three events together do shed some light on is what privileged tech types (myself included) believe is important. And one really interesting trend has emerged: it would seem this select audience believes that AI is no longer cool. AI is yesterday’s news, having been replaced by discussion of other cutting-edge “disruptive” technologies like quantum computing and biotech.
This is a significant change from my last foray on the in-person tech circuit in late 2019 to early 2020, when AI was the thing on everyone’s lips. So, what’s changed?
AI is widely available
First, AI and the broader set of data-driven technologies it forms a part of, is now seen as ubiquitous. Indeed, just about every session across the three conferences I attended (and the sessions I presented or was on panels for) included mention of data as part of the agenda.
AI and other data-driven technologies are everywhere. They have accelerated so much since 2019 that every key industry has either deployed AI (mostly different flavors of machine learning) or is in the process of doing so. We heard from CogX co-panellist Maria Axente, AI for Good Lead at PwC, a persuasive argument that we are seeing the rapid commoditization of the AI approaches developed over the past decade, moving them from R&D to off-the-shelf products and services.
This is a significant change. When I founded the first data science team at the Wellcome Trust in 2017, it was after having tested the top off-the-shelf AI products then available—mentioning no names—and finding them still immature for implementation. So, instead, we built an internal R&D team in Wellcome and developed our own bespoke AI tools. Clearly, that age of bespoke development is quickly coming to a close, at least for common, broadly applicable tasks. The off-the-shelf AI tools have dramatically improved and the prices have dropped sufficiently to now make developing bespoke tools less economical, particularly considering the huge rise in salaries for top data science talent.
AI research has narrowed
The maturity of AI is closely tied to a second big change: the range of actors doing cutting-edge AI research has drastically narrowed, and so has the breadth and diversity of subjects and applications being researched in the field of AI. Another great expert on our CogX panel was Juan Mateo-Garcia, who leads the Nesta data team. Juan and his co-author published an insightful paper a while back that showed this very trend: A Narrowing of AI research? —though I fear now there is no need to include the question mark at the end of that paper’s title.
The dynamics are simple to understand, though no less worrying. Over the past decade, we have seen an accelerating brain drain of top AI researchers leaving academia to work at the world’s top tech companies. The reason is not just the much higher salaries the tech companies are able to pay; at least as important, if not more so, is the availability of computational resources. Many of the most popular AI approaches of the past few years are enormously computationally hungry and universities are simply unable to provide the supercomputers or cloud infrastructure needed to train many classes of models, such as Large Language Models (LLMs). And, unsurprisingly, once AI researchers are paid by the tech companies their research narrows to align with the interests of the companies, resulting in more research being done in fewer areas.
This was a very lively topic of discussion at SciFoo, where top AI researchers still in academia and those now in the tech sector were both well represented. This trend and its negative consequences were acknowledged by both camps. By hollowing out academic AI research and narrowing the focus, we are closing off potential avenues of future innovation: put plainly, we are in danger of sacrificing the future of AI for its present. Everyone agreed this was bad, but it was harder to come up with workable solutions. Suggestions ranged from taxing big tech more and investing those funds in strengthening basic research in academia to big tech proactively investing more in supporting the academy, but without strings attached, to ensure academic freedom is preserved. Personally, I believe the problem is serious enough that we need both approaches at the same time.
Enter impact data science
This brings me to Aspen, where I was invited by Mastercard Center for Inclusive Growth to present on the topic of how to develop the field of impact data science: i.e., the application of data science to social impact issues such as health, climate, financial inclusion, and social justice. The argument I was making at Aspen was that we need to train a new generation of data scientists for the social impact sector, more diverse and equipped with broader, more interdisciplinary skills, as called for in data.org’s recent report Workforce Wanted: Data Talent for Social Impact.
The current trend of both increasing commoditization of AI and narrowing of AI research makes an intentional focus on how we train data scientists for the social impact sector all the more urgent. Today, off-the-shelf AI products and services have not been designed with the social impact sector in mind. The typical use case for these AI products is retail or marketing; crucially, the data their algorithms are trained on is significantly different from health, climate, or community engagement datasets required to solve many of the most burning social impact challenges affecting the world.
Using these existing AI solutions in a social impact context would be fitting square pegs into round holes, introducing biases, and leading to inappropriate conclusions. To maximize the chance of success in the social impact sector, we need tools designed for specific contexts and that means data scientists and data engineers knowledgeable not just in technical areas such as Python or data pipelines, but also in the subject matter of particular expert domains such as health, climate, financial inclusion and social justice. They need to understand the fragmented, uneven and unrepresentative state of the data and must have the skills to engage with often vulnerable communities who, for good reason, start from a position of low trust.
On the positive side, the range of data science and AI approaches useful for the social impact sector is much broader than the currently dominant domains in the tech industry and many/most don’t require computationally heavy deep learning. So, there is an opportunity in the current state of affairs to reinvigorate academic research in data science and AI by funding more foundational research, teaching, and training in interdisciplinary areas more relevant to social impact sector.
This work should include areas such as sound data governance and data stewardship practices that center concerns of privacy and equitable data ownership, de-colonized and non-extractive data collection practices, participatory design grounded in understanding cultural context, ethics, and long-term social impact analysis integrated into the product life-cycle (here is a paper we published about this kind of interdisciplinary intervention that I presented at SciFoo: Integrating Ethics into Data Science: Insights from a Product Team). It should also incorporate more subject matter-relevant skills for data and AI in public health, climate science, financial inclusion, and social justice. There is a rich tapestry of new research fields and applied skills waiting to be developed.
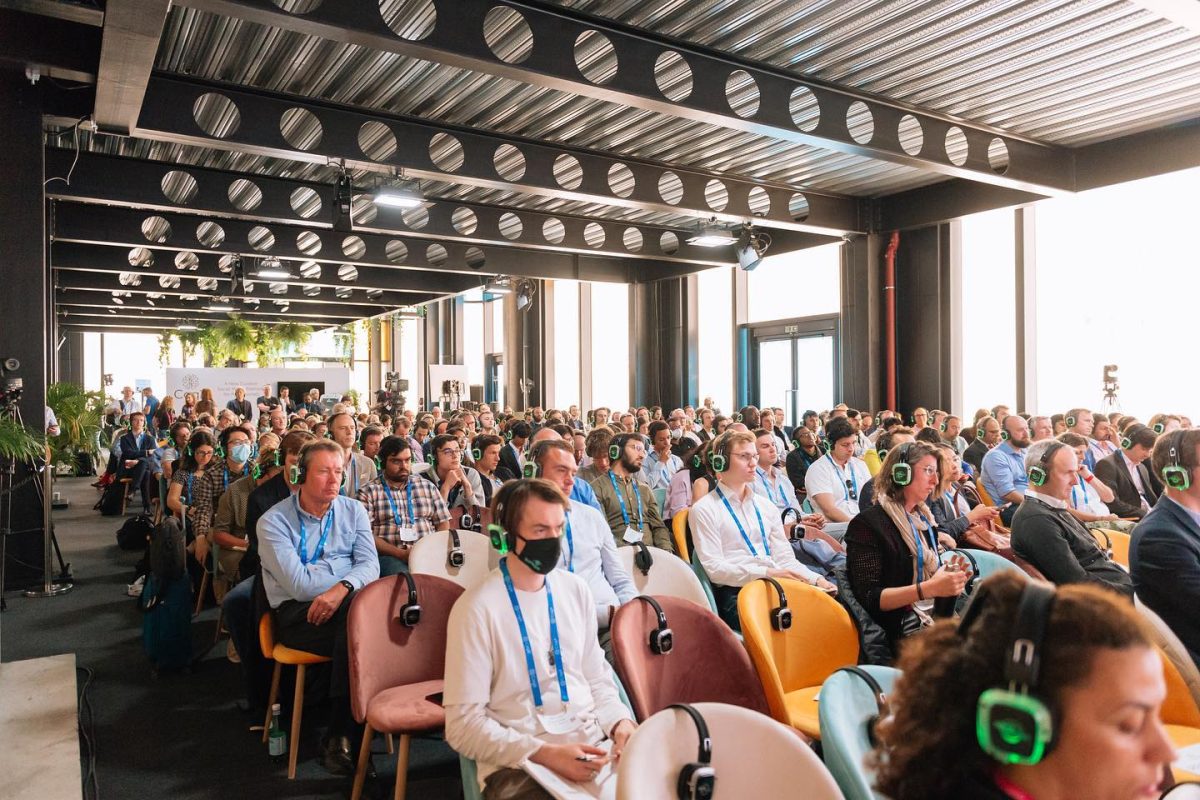
Doing so will draw more – and more diverse – students back into data science research and practice outside of big tech, equip the social impact sector with new talent, and broaden again the range of AI research that will benefit everyone (big tech included). We at data.org believe that strengthening talent and application for social impact present an opportunity to strengthen the entire AI ecosystem – and are committed to doing our part to drive that impact forward.
About the Author
Danil Mikhailov, Ph.D. is the Executive Director of data.org. He has over 20 years of experience setting up multiple start-ups and leading work across a range of diverse sectors, always investigating and innovating in the space where technology, culture, and society converge.
Read more