This piece is the culmination of a series of explorations seeking to map and make sense of the landscape of efforts to apply data for good. The process involved not only a review of the field, but also a taxonomy for understanding how different initiatives labeled as “data for good” or “AI for good” relate to one another. This work represents input from over 30 stakeholder interviews and six live feedback sessions. This article is the final iteration of this work, but previous updates were published in parts 1 and 2 of this series, if you’d like to see the evolution of the landscape.
There is huge potential for AI and data science to play a productive role in advancing social impact. However, the field of “AI for good” and “data for good” is not only overshadowed by the public conversations about the risks rampant data misuse can pose to civil society, it is also a fractured and disconnected space without a single shared vision. One of the biggest issues preventing AI for Good from becoming an established field is a lack of clarity. “Data” and “AI” are treated like they’re well-defined terms, but they are stand-ins for a huge set of different – and sometimes competing – outcomes, outputs, and activities. The terms “AI for Good” and “Data for Good” is as unhelpful as saying “Wood for Good”. We would laugh at a term as vague as “Wood for Good”, which would lump together activities as different as building houses to burning wood in cook stoves to making paper, combining architecture with carpentry, forestry with fuel. However, we are content to say “AI for Good”, and its related phrases “we need to use our data better” or “we need to be data-driven”, when data is arguably even more general than something like wood.
One way to cut through this fog is to be far more clear about what a given AI-for-good initiative seeks to achieve, and by what means. To that end, we’ve created a taxonomy, a family tree of AI-and-Data-for-Good initiatives, that groups initiatives based on their goals and strategies. This taxonomy came about as a result of studying and classifying over 600 initiatives labeled as “AI for good” or “data for good” publicly (you can read more about the methodology here). At the highest level, the taxonomy identifies six major branches of AI and data for good activities, which will help field builders, funders, and nonprofits better understand the distinctions in this space. Within each branch, you’ll see sub-branches that further subdivide initiatives, which we hope will aid collaboration for the groups within those branches and help funders make more strategic funding decisions.
Below you’ll find a landscape map of a subset of initiatives in the AI and Data for Good space, categorized into those six main branches we observed. Before we dive into describing each branch, here are a few caveats on how to interpret this work:
- We are focused on initiatives that are enabling and advancing the field of Data for Good, not trying to catalog every data science project every nonprofit or company is doing (though that would be an interesting landscape to see as well). These initiatives are those focused on advancing the field of using data and AI for good.
- We chose to categorize initiatives, not organizations. It would be impossible to classify “Microsoft”, when their activities span funding through Microsoft Philanthropies, creating open satellite imagery through Microsoft AI for Earth, providing infrastructure through nonprofit licenses of Microsoft Azure, and so on. Therefore you may see organizations appear multiple times across the landscape.
- The current database of organizations is minuscule – it is nowhere near exhaustive. We have only mapped enough initiatives to show the results for feedback, but we encourage you to recommend other initiatives you know of but don’t see here with this form.
- While we are incredibly privileged to have advisors contributing to this project from countries like Brazil, Nigeria, and Nepal, the initiative still skew Western.
You can contact the author with any feedback at jakeporway@gmail.com .
Enjoy!
Acknowledgments
I want to thank everyone who signed up to be an advisor on this project, as well as those who were willing to give me some of their time to craft this first version of the landscape. Huge appreciative thank yous to Olubayo Adekanmbi, Aman Ahuja, Carol Andrade, Afua Bruce, Peter Bull, Kriss Deiglmeier, Mariel Dyshel, Chapin Flynn, Matt Gee, Josh Greenberg, Elizabeth Grossman, Mark Hansen, Perry Hewitt, Priyank Hirani Brigitte Hoyer Gosselink, Claudia Juech, Zia Khan, Tariq Khokhar, Juan Mateos Garcia, Andrew Means, Danil Mikhailov, Josh Nesbit, Craig Nowell, Ben Pierson, Uttam Pudasaini, Giuilio Quaggiotto, George Richardson, Michelle Shevin, Sarah Stone, Evan Tachovsky, Jenny Toomey, Stefaan Verhulst, Sherry Wong, Chris Wiggins, Chris Worman, Ginger Zielinskie
The Landscape of AI and Data for Good
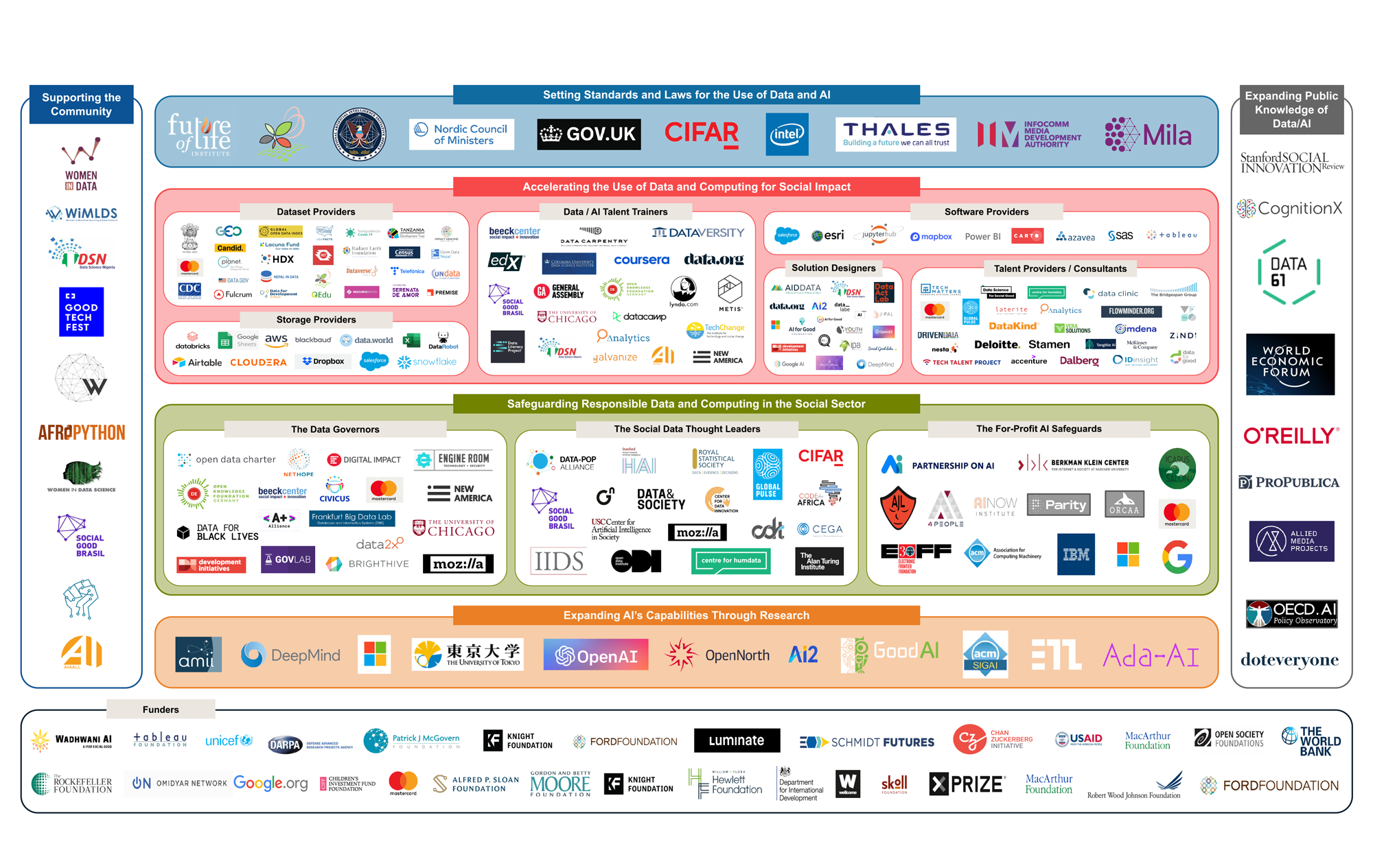
What you see above is a landscape of initiatives that are doing “AI/Data for Good”, grouped by the six major branches they fit into. Some salient sub-branches are pictured as well. While the map does not depict every initiative and sub-branch, it offers a good enough understanding of how the field is divided. Let’s talk about the high-level branches of AI/Data for Good and identify some of their key sub-branches.
Related Article
Setting Standards and Laws for the Use of Data and AI (The Regulators)
Mission and Strategy: We set the laws and standards for how data and AI can be used, legally speaking. We often operate at a global level, be that international, national, or across a field. Our decisions affect every type of system.
- This family of initiatives sets the rules for how AI and data can be used through regulation and standard setting. They are often (but not always) government institutions. They do not usually have a specific agenda in terms of harm reduction or creation of data solutions. Instead they tend to take holistic views of data and AI and set strategies that balance innovation with protection. For example, laws and strategies originating from AI.gov often speak of protecting personal data collected by companies and fostering more robust AI innovation in the same breath.
- In the second layer, we note that some policies target specific parts of the data pipeline, such as data collection or curation, while others tackle the outputs or uses of AI specifically. In our taxonomy this camp serves its purpose by ending at this resolution, but it would be served well by a deeper taxonomy that further classifies types of laws and policies in future work.
- Examples: White House Office of Science and Technology Policy, The European Group on Ethics in Science and New Technologies (EGE), The Centre for Data and Ethics Standards.
- Sub-branches
- Within the regulators, we only observed one more useful level of branching, which divides regulation up across the AI / DS pipeline: regulation of AI inputs, regulation of AI outputs, and regulation of human use of AI. This is due in part to regulation being so wide-ranging. Most regulation covers many different interventions, so at most we saw distinction in which part of the AI / data science pipeline they focused on. In our taxonomy this camp serves its purpose by ending at this resolution, but it would be served well by a deeper taxonomy that further classifies types of laws and policies in future work.
Expanding AI’s Capabilities Through Research (The AI Researchers)
Mission and Strategy: We use fundamental technological research as our strategy for making change. Our definition of “for good” focuses mainly on encoding ideas of fairness and equity into computer code itself.
- This family of initiatives determines the power and capabilities of data and AI. Their theory of change is that, by adjusting the technical capacity of AI, they can ensure it is “safe”. This family of initiatives tends to spring from research universities and corporate research labs who believe that “fairness” can be encoded. They end up determining what data, computing, and AI are technically capable of, modulated by the laws set forth by The Regulators.
- In the second layer of resolution, we simply note that some initiatives specifically target different stages of the pipeline. The vast majority of these efforts focus on the modeling and output phase. There are a few notable exceptions, such as Differential Privacy, which focuses on the data curation phase, allowing machines to draw inferences from personal data without necessarily ever viewing or sharing the underlying data.
- Note, these are not researchers who are studying the impacts of AI or think tanks reflecting on the implications of AI, like AI Now or Data and Society. These are explicitly technological researchers who are using software as their key intervention.
- Examples: OpenAI, Allen Institute for AI, Deep Mind, Microsoft Research Lab
- Sub-branches
- Within the AI Researchers we saw very little branching. Most research was focused on innovating on the core algorithms in AI, like changing the way language models were learned, or creating new outputs, like “fairer” models of face recognition. There were some efforts focused on the creation of AI, such as creating chips that had smaller environmental footprints, but they were in the minority. Therefore, like the regulators, we only branched this group by one level to identify the coarse parts of the pipeline they sought to change.
Note that The Regulators and AI Researchers encompass the inner groups in our landscape map because they are the two groups that set the standards by which all other groups must abide. The AI Researchers expand the capability of computers while the Regulators limit how it can legally be used by humans. Their decisions affect almost every system, and they do not exclusively focus, at this resolution, on harm reduction or increasing application.
Our next two camps occupy the center of the landscape and are invested in more direct social impact outcomes.
Related Article
Accelerating the Use of Data and Computing for Social Impact (The Solution Creators)
Mission and Strategy: We seek to apply data and computing technology to problems with social outcomes. Our strategy for doing so is reducing the cost and time it takes for these solutions to be built.
This family of initiatives seeks to apply data science to more social issues by reducing the barriers to create data solutions. Their theory of change is that, by providing data science capabilities or directly creating data science solutions themselves, more social issues will be mitigated.
Examples: CDC datasets, free storage from Salesforce, Tableau software, Galvanize data science training, McKinsey Noble Intelligence, DrivenData, data.org’s Epiverse pandemic tools
Sub-branches
Initiatives in this branch tend to focus on one of two sub-strategies: creating more data science inputs for the social sector to use or directly creating more data science outputs for the social sector. Within the strategy of creating more data science inputs, we see initiatives focus on four inputs to the data science pipeline: some initiatives help identify and scope appropriate problems, some provide new data or data collection techniques, some provide services and products to store and curate data, and others create more data science talent through training and cultivation. The hope from these products and services is that a greater and more accessible supply of these inputs will make it more feasible for organizations to apply data science to social issues.
The initiatives focusing on creating data science outputs directly tend to take of one of three forms:
- They create DIY software: These initiatives build software that other organizations can use to build their own data science outputs. Examples: Tableau, AutoML
- They create off-the-shelf solutions: These initiatives build final products that others can utilize. These outputs could be analyses, statistical models, or software. Examples: Facebook Data for Good products, JPAL statistical models
- They provide consulting services: These initiatives partner with SIOs to understand their data needs and provide short-term capacity to meet them. Examples: DataKind, McKinsey Noble Intelligence, DrivenData
It is worth noting that, even within these three categories, initiatives may provide services and products that meet one or more of the three data science outcomes Observe, Reason, and Act (for definitions of these AI/data outcomes, please see our article on the Three Uses of Data). This distinction can be valuable when assessing the types of services available in the market. For example, DataKind will staff volunteers to help SIOs solve any of Observe, Reason, or Act. IBM’s Science for Good, by contrast, only takes Act-type problems. IDInsight and JPAL, specializing in measurement and evaluation, primarily provide solutions to Reason-type problems.
There is one last group of initiatives in this branch of the tree, the Data Strategists. These initiatives seek to set strategy and/or culture within SIOs so that, ostensibly, they can make more impact. They are a separate class of initiatives from the solution creators because they do not necessarily target one stage of the data science pipeline, nor do they create a product. They instead intervene to build an SIO’s capacity.
Safeguarding Responsible Data and Computing in the Social Sector (The Safeguards of Society)
Mission and Strategy: We seek to reduce harms that arise because of applications of data and AI technology. These harms are usually human rights offenses or challenges to environmental sustainability.
- This family of initiatives seeks to reduce harms to people and the environment as a result of misuse of data and technology. Their theory of change is that, by changing data science practices along the data science value pipeline, they can create more ethical outcomes that preserve human rights and environmental sustainability.
- Examples: AINow, Algorithmic Justice League, Data Pop Alliance, UN Global Pulse consulting, The Engine Room, GovLab, Google+Microsoft’s Model Cards, programs that teach ethics to engineers.
- Sub-branches:
- The two broad sub-strategies in this family focus on reducing harms within the for-profit and not-for-profit sectors. Like all of our sub-branches, these efforts are divided into the stages of the data science pipeline they seek to affect: the inputs and activities, the outputs, or the end-user use. Like the Data Strategists in the Solution Creators branch, there is also an Ethical Strategists family of initiatives that seeks to increase an organization’s ethical capacity and/or design more ethical strategies for them. What is key to note is that the efforts used by each group depend heavily on whether they’re trying to affect the systems of for-profit or not-for-profits (which have differing incentives) and whether they’re operating within the system or without it. This difference can be summarized in the following table:
For profit | Not for profit | |
---|---|---|
Working from outside the system | Reducing harms from tech companies using advocacy and regulation (e.g., Algorithmic Justice League) | Advising SIOs on best practices using research and advocacy (e.g., Data Pop Alliance) |
Working from inside the system | Reducing harms from tech companies using changes to internal practice (e.g., Google’s Model Cards) | Utilizing the best practices for designing data-driven solutions within a non-profit (e.g., IDInsight applying ethics when building a model for a client) |
The next two groups bookend the landscape, because they do not have explicit harm reduction or application goals, nor do they focus on a specific sector. They exist to strengthen a capacity in society, and thus are often included in lists of organizations doing “AI for Good” or “Data for Good”. Their definitions of “good” are quite general: increasing representation and happiness within the AI profession, and educating the public on any topic about AI or data science.
Supporting the Community (The Community Builders)
Mission and Strategy: Wherever there are AI or data experts, we seek to strengthen their satisfaction within the profession by increasing representation, helping them learn new skills from classes and from one another, and forming community platforms on which they can convene.
- This family of initiatives seeks to strengthen the data science and AI practitioner community. Their theory of change is not explicitly about creating new solutions or reducing harm (hence their position outside of the center circle). Instead, they have an intermediate human rights goal of either increasing representation and access to the profession of data science, or simply enriching existing data scientists through shared community.
- Examples: Black in AI, Lesbians Who Tech, Women in DS and ML, Good Tech Fest, Social Good Brasil
- Sub-branches:
- Here we did not branch further than the efforts to build communities. As they don’t seem to be angling for a specific social outcome or a change to any part of the data science pipeline outside of talent, nor are they using many activities outside of community organizing, this group stands out as a terminal node in and of itself.
Expanding Public Knowledge of Data/AI (The Journalists)
Mission and Strategy: We seek to write publicly about the latest innovations and risks of data science and AI so the average citizen can understand more about this technology.
- This family of initiatives reports on the activities of the data science and AI communities. The Journalists do not have a crisp theory of change in the terms of our taxonomy, as they rarely explicitly seek to reduce harm or increase application of DS. Instead, they seek to inform audiences about the latest innovations, challenges, and practices in the space in an effort to combat the structural lag between fast-moving uses of data and public understanding. In this way they are often like the Safeguards of Society, but due to the flexible nature of their reporting and lack of an advocacy agenda, they are in a different class. Like the Community Builders they are outside the circle as they are not actively trying to apply DS or change its application. While certain outlets have different bents for different audiences, these initiatives are characterized by broad reporting on the DS for Good space.
- Examples: SSIR, O’Reilly Media, World Economic Forum, Gartner
- Sub-branches
- Like the Regulators, this group is often too diffuse to separate into specific theories of change or activities on specific parts of the data pipeline. This is another family that others could expand with a specific taxonomy of data and AI related media.
Funders: We have listed funders separately for ease of viewing in the Compressed version. However, each funder’s initiatives would fall within one of the other camps according to what activities and strategies they are funding.
Takeaways and Closing
Looking at the landscape as it is today, we noticed a few aspects of the field that are worth naming explicitly:
- The family of services seeking to accelerate the use of data and computing in the social impact space is wildly fractured. Lots of companies provide short-term services to the social sector, but do so at varied points along the data science pipeline. This fragmentation means that nonprofits struggle to build data science capacity or create new data science projects without a way to navigate these resources and cobble them together into something meaningful. The field could benefit from someone providing a directory of services and tips on how to engage with them appropriately. At a minimum, an organization that helps guide people through the field, akin to what data.org is doing with their Resource Library, would be hugely helpful.
- The debate about data ethics and AI ethics is raging, but the conversation is often without the context of the systems each actor is working in. However, nonprofits, governments, and for-profit entities operate under different incentive structures and with different cost-benefit structures to their actions. For example, we know that collecting race data can often do more harm than good, but there are hugely different implications of an ad-targeting company collecting race data to optimize ads versus a nonprofit research center collecting race data to create more equitable health treatments. This observation is not to imply the not-for-profit sector is always doing good with data or doesn’t need to think about ethics, or that every for-profit entity is misusing data. Far from it. We hope instead that people augment their conversation about appropriate use of data and algorithms with specificity about the type of behaviors appropriate with that system and a keen eye for unintended consequences, under those contexts.
- We notice a lack of organizations that exist to tackle a social problem at scale. Much of the data for good field is focused on either creating individual solutions for individual organizations or developing general capacity. We believe that a third strategy focused on identifying key areas of opportunity for data and computing within a systems context is needed.
With this taxonomy, we hope that funders, field builders, and social impact organizations in the field will be able to navigate the variety of different Data/AI for Good initiatives and more strategically align their efforts. We hope this landscape has provided a useful framework for consideration, and welcome your expansions and contribution to this work.
About the Author
Jake Porway loves seeing the good values in bad data. As a frustrated corporate data scientist, Porway co-founded DataKind, a nonprofit that harnesses the power of Data Science and AI in the service of humanity by providing pro bono data science services to mission-driven organizations.
Read moredata.org In Your Inbox
Do you like this post?
Sign up for our newsletter and we’ll send you more content like this every month.
By submitting your information and clicking “Submit”, you agree to the data.org Privacy Policy and Terms and Conditions, and to receive email communications from data.org.