More than 50% of nonprofits report that their organization uses generative AI in day-to-day operations. We’ve also seen an explosion of AI tools and investments. 10% of all the AI companies that exist in the US were founded in 2022, and that number has likely grown in subsequent years. With investors funneling over $300B into AI and machine learning startups, it’s unlikely this trend will reverse any time soon.
Not surprisingly, the conversation about Artificial Intelligence (AI) is now everywhere, spanning from commercial uses such as virtual assistants and consumer AI to public goods, like AI-driven drug discovery and chatbots for education. The dizzying amount of new AI programs and initiatives – over 5000 new tools listed in 2023 on AI directories like TheresAnAI alone – can make the AI landscape challenging to navigate in general, much less for social impact. Luckily, four years ago, we surveyed the Data and AI for Good landscape and mapped out distinct families of initiatives based on their core goals. Today, we are revisiting that landscape to help folks get a handle on the AI for Good landscape today and to reflect on how the field has expanded, diversified, and matured.
Related Article
A Quick Look Back
When we last charted the landscape, we found it helpful to categorize “Data/AI for Good” groups by the different philosophical outcomes they aimed to achieve. Some wanted to supercharge nonprofits with data science, while others sought to safeguard society from biased and unethical applications of machine learning. Others concentrated on training and nurturing new data scientists or funding the entire ecosystem. As we explore the landscape again, we see that many of these founding ideas remain consistent, and in this AI revolution, trust, investment, and skilling in data cannot be left behind. Data is the underpinning of AI and will continue to be the critical building block for the AI infrastructure. However, there are two main changes we observed:
- In the first landscape, all the programs we surveyed focused on supporting the field of data and AI for good. We didn’t focus on many examples of people using data science for good in their work because the list would have been enormous. Today, however, we are still early in our understanding of the ways AI can support social impact. Therefore, we are highlighting some example organizations across domains that are using AI for social impact.
- In the first landscape, there were not many consumer products for data science and AI that we would mention. Data visualization tools like Tableau or machine learning platforms like AWS Sagemaker existed, but they still largely required technologists to run and were intended for technical tasks. In this wave of AI, we have seen a wave of consumer software, from general Large Language Models (LLMs) like ChatGPT to AI-ified programs like Salesforce. In this landscape, we name some examples of off-the-shelf AI software that nonprofits could use to illustrate the difference between off-the-shelf AI tools and custom AI solutions.
The Five Branches of AI for Good
First, let’s discuss the taxonomy we used for categorizing AI for Good programs. In reviewing a wide array of current AI for Good programs, we noticed they tended to fall into five main branches:
- Creating More AI for Good: This category includes initiatives that focus on expanding the ecosystem’s ability to build AI solutions for social impact. They often provide one or more of the following:
- Data: Open data catalogs like the Humanitarian Data Exchange (HDX) or Radiant Earth Foundation’s MLHub feed nonprofits, researchers, and innovators with clean, relevant datasets that can be used to train AI models for social impact.
- Cloud: In order to store all of the data needed for AI, cloud providers like AWS and Google have provided free storage and cloud credits to nonprofits and mission-driven organizations. In addition, very few organizations will have the local infrastructure needed to build and run AI models, so cloud providers like Amazon Web Services or Google Cloud Platform provide a wide breadth of online services that nonprofits can access at free or discounted rates to train and deploy AI.
- Compute: Tech companies like Google and NVIDIA have made some limited discounts on costs for GPU usage for nonprofits, while initiatives like Empire AI plan to provide GPUs to researchers in New York, so they can train and run models more cost-effectively.
- Models: Projects like BigScience’s BLOOM or Hugging Face’s Hub Community Models offer open-source AI models ready for fine-tuning by nonprofits and government agencies.
- Expertise: Groups like Zindi Africa and Tech to the Rescue connect AI professionals with social-sector organizations that do not have those technical skills in-house.
- Guidance and Frameworks: Programs such as OpenAI’s AI Academy or NTEN publish frameworks, tutorials, and practical resources to help nonprofits design, build, and acquire AI solutions to meet their mission.
- Funding: Grantmakers like Google.org’s AI Impact Challenge or the Mastercard Center for Inclusive Growth provide financial support to nonprofits, startups, and researchers working on social impact AI projects.
- Building Skills to Create More AI for Good: Another group of initiatives invests in capacity development through building skills and knowledge across diverse communities. They often offer workshops, online courses, mentorship programs, and in-person training. Programs like TechChange’s AI and Machine Learning Courses for Social Good, Fruit Punch’s AI for Good Challenges, and Data for Development’s ChatGPT Trainings target everyone from high school students to seasoned professionals looking to pivot into AI for social impact. This educational dimension is critical since nonprofits and grassroots organizations need to grow in-house capacity to design, implement, and maintain AI solutions that truly match their missions.
- Ensuring Responsible AI: As AI has become more prevalent, so too have concerns about issues like bias, privacy, and accountability. Indeed, one of the biggest shifts we’ve observed since our initial mapping is the growth of efforts dedicated to governing AI responsibly. Mozilla Foundation’s Trustworthy AI Initiative, IEEE’s Global Initiative on Ethics of Autonomous and Intelligent Systems, and the research from AI Now Institute represent some of the many groups providing ethical frameworks, research, and policy guidance. These organizations underscore the notion that “for good” cannot merely mean deploying AI anywhere but deploying it in a way that safeguards human rights, fairness, and transparency. They tend to provide frameworks and guidance on ethical practices, with a few groups, like ORCAA Consulting, providing ethical AI audits.
- AI Software for Nonprofits’ “Back Office”: Beyond programmatic uses of AI, there is also a growing demand for solutions that streamline nonprofit operations, communications, human resources (HR), fundraising, and data management. Platforms like Textio help reduce bias in job postings, DonorSearch AI offers donor scoring for fundraising, while AI-driven CRMs from Salesforce.org and Keela aim to maximize fundraising efficiency. Although these “back office” tools do not always directly tackle a programmatic challenge (e.g., health, education, or climate), they play a crucial role in saving nonprofits time and money, which can be redirected toward mission-critical work. We wanted to name this set of programs and software as its own branch in the taxonomy to distinguish it from custom AI solutions that nonprofits build for mission delivery and to acknowledge that most nonprofits will experience this type of AI before any other.
- Demonstrating AI Use in Key Issue Areas: As mentioned above, AI is so new that we felt it would be useful to highlight a few organizations that are applying AI to their program delivery as examples for the sector. We’ve selected a few examples in categories that data.org focuses on:
- Health: Jacaranda Health applies AI-assisted tools in developing healthcare infrastructures, while Google DeepMind’s AlphaFold fosters scientific breakthroughs in protein folding and drug discovery.
- Financial Inclusion: Grameen Foundation uses AI to help underbanked folks feel comfortable accessing capital the way they want to and microloan platforms like Tala analyze mobile phone data to offer small lines of credit to unbanked populations.
- Climate: Climate TRACE uses AI to track greenhouse gas emissions globally, SilviaTerra helps optimize forest management, and Earthshot Labs models ecosystem restoration.
- Education: Tools like Khan Academy’s Khanmigo offer AI-based personalized learning, while Amira Learning helps young readers practice literacy skills.
- Food: Chatbots like Digital Green’s Farmer. Chat provides customized support to farmers while programs like Zero Waste Zero Hunger use AI to 3D scan and image discarded food to quantify food waste.
- Gender: Initiatives such as Myna Mahila Foundation’s AI Chatbot and Girl Effect’s sexual health chatbots address issues ranging from women’s health to girls’ education barriers.
These targeted projects serve as exemplars, real-world demonstrations that AI can improve social outcomes when properly tailored and thoughtfully implemented. This list highlights a few of the many impactful initiatives in this space.
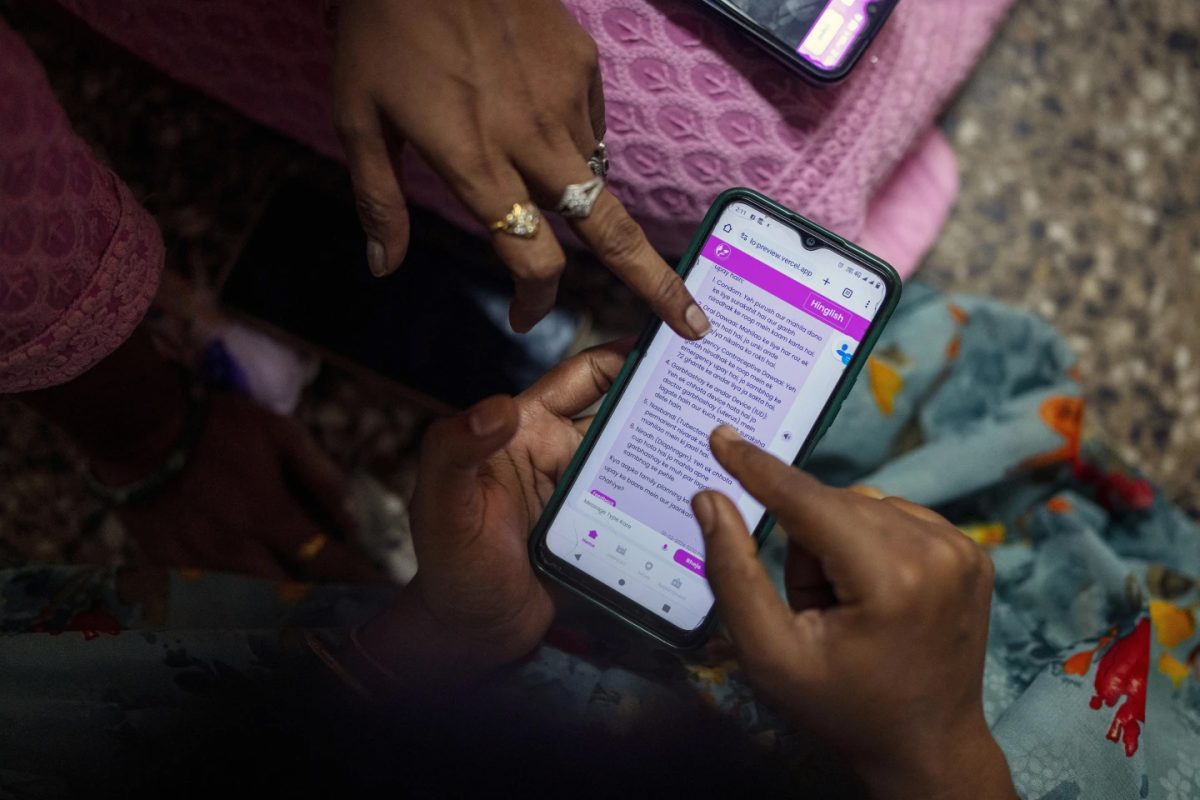
Trends and Observations
While these five branches capture where AI for Good is today, they also reveal new opportunities and challenges:
Generative AI is on the rise, but don’t forget “traditional” AI/ML
The organizations in our landscape serve a mix of Generative and non-Generative AI needs. That distinction is worth noting – there is still much value in traditional AI/ML approaches, such as prediction and classification. Many of the data and service providers, like AWS, are still necessary for driving innovation. What we observe is that more organizations are trying new Generative AI tools, because they are making their way into consumer products and are low barrier to entry. However, for custom in-house work, more sophisticated, but traditional, methods are needed. This finding aligns with observations from the Giving Tuesday AI Readiness assessment in nonprofits, which found that 70% of “high AI use” nonprofits reported using Generative AI, while 30% of that same group reported using AI tools for data organization and interpretation, and 20% for prediction.
Early Prototyping Is Easier, but Implementation is Hard
In our research, we found that many nonprofits can take advantage of some of the new AI software available or AI 101 training to create powerful proof-of-concept AI models for their work. We see many initiatives designed to help nonprofits with this stage, offering them data, training, and models. However, moving from prototype to deployment still poses challenges, from insufficient budget for ongoing maintenance to a lack of dedicated AI staff who can iterate and refine solutions. There are very few if any, programs or initiatives at this stage that help organizations move past a ChatGPT prototype to a working piece of software. This “implementation gap” highlights a significant need for support services that take an AI solution from demo to an operational reality in the nonprofit context.
Back Office vs. Programmatic AI
As mentioned above, AI in nonprofits often falls into two categories: improving back office processes (like HR, and donor management) or driving mission-related outcomes (like tracking deforestation or diagnosing diseases). The skills, tools, and training needed to adopt AI in these two domains can vary significantly. We see a developing divide in the marketplace between solutions built for operational efficiency for common operations and those aimed at a nonprofit’s unique programs. Philanthropy will need to develop ways for nonprofits to get AI support for both types of workflow.
Problem Identification Is Key
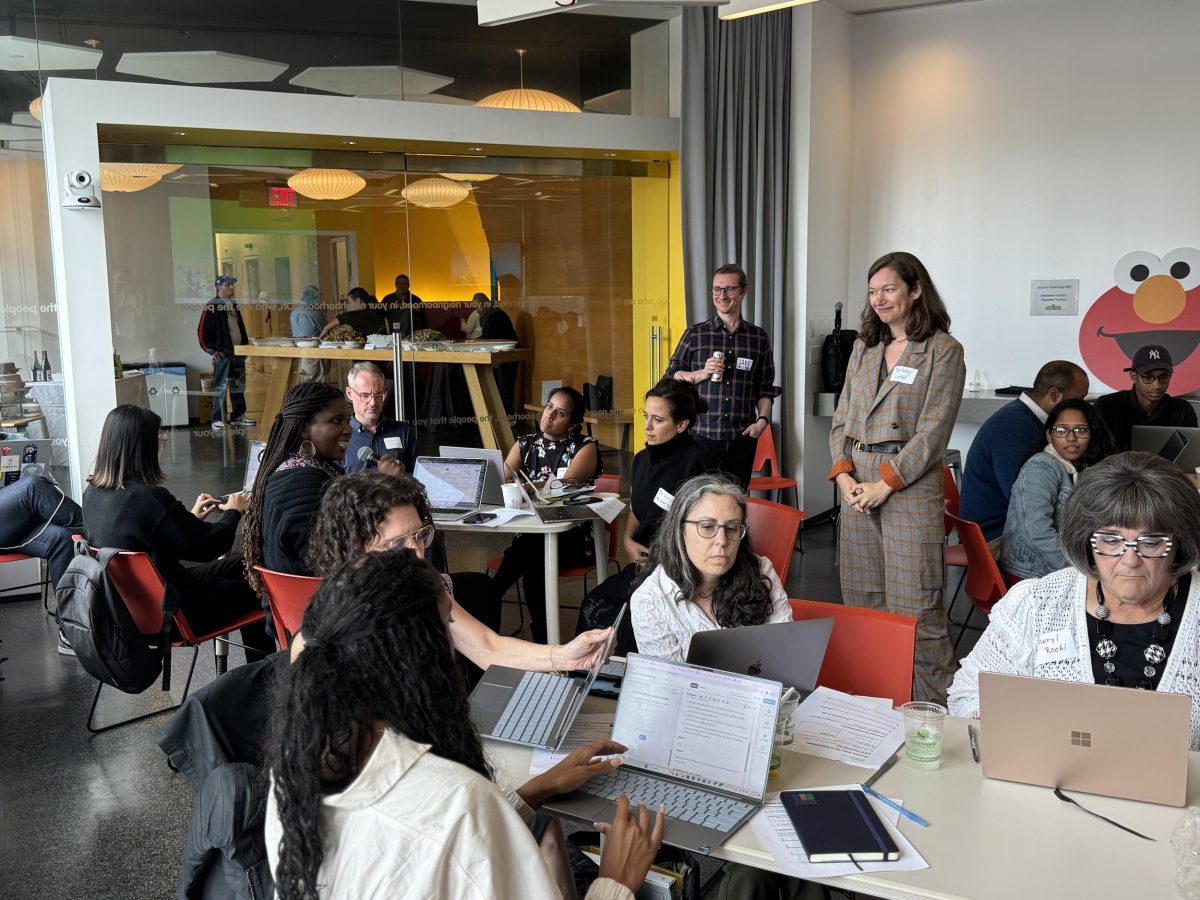
One assumption many AI for Good programs make is that nonprofit partners already know which problems are ripe for AI intervention. In practice, identifying the right AI problem, where data is available, where impact is feasible, and where technology can truly address the root causes, requires specialized expertise. We need more consultative or discovery-focused services, like Decoded Futures – a program that helps nonprofits and technologists in NYC collaborate to identify AI-driven solutions – in this space to help mission-driven groups pinpoint where AI can add the most value.
The Rise of Responsible AI Awareness
Since our previous landscape analysis, the sector has made real strides in recognizing the importance of responsibility, bias mitigation, and inclusive design in AI. Numerous new initiatives, think tanks and policy groups are shaping robust guidelines to ensure AI serves humanity in equitable ways. This progress is encouraging, yet the field could still benefit from practical mechanisms that hold organizations accountable to principles that can be easily adopted and implemented.
Success Stories Need Common Evaluation Standards
Showcasing success stories is powerful, but many new AI for Good efforts lack clear metrics for measuring outcomes or calculating return on investment, especially in this early stage. Without rigorous and mutually agreed upon evaluation frameworks, it is difficult for funders, nonprofits, and governments to understand which interventions have the greatest social or environmental impact. Consequently, it remains challenging to rally large-scale funding or mobilize broader adoption. The landscape will need to develop more evaluation resources or organizations to move forward.
Where Do We Go From Here?
The rapid acceleration of data and AI for Good has yielded a new wave of thoughtful practitioners and some promising returns, but significant gaps remain. To address these, we recommend the following steps forward:
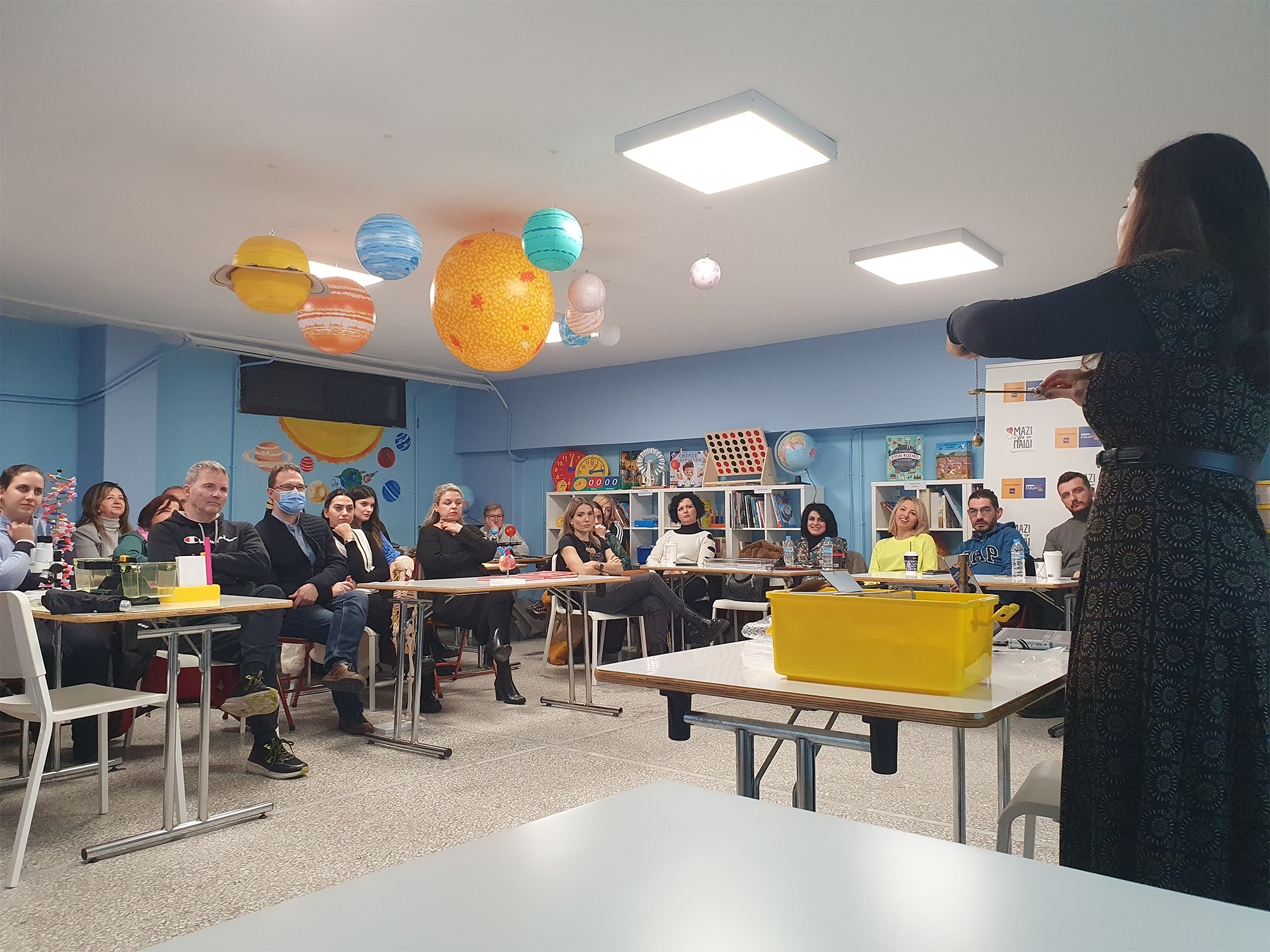
Filling the Evaluation Gap
It is currently difficult for social sector organizations to assess in advance how much impact AI will have for them, making it difficult to justify the investment. More robust evaluation and estimation models could solve this. Philanthropy could fund the creation of these metrics and frameworks. Tech companies as well could help by sharing their evaluation frameworks for their models and helping social sector organizations apply them to their work.
Cross-Sector Learning
The AI for Good field needs more robust knowledge-sharing across sectors. Whether it’s government, philanthropy, nonprofits, academia, or industry, each brings unique expertise and data. Encouraging multi-stakeholder forums, hands-on workshops where technologists and nonprofits work together, or peer-learning communities can accelerate solutions that meet real community needs. Tech companies also have a unique role to play in this solution by offering any guidance on best practices, be that in model selection, model use, or ensuring AI alignment and safety.
Increasing Resourcing
There is no doubt that one of the biggest bottlenecks to deploying AI in the social sector is resourcing. Philanthropic funders can increase the supply of flexible and experimental funding so nonprofits can experiment with AI. Technology companies can play a leadership role in providing discounted or pro bono versions of their models and services to nonprofits. They can also create or join programs to donate their technical staff’s time to support nonprofits in identifying and solving their AI problems or setting up the most efficient infrastructure for their needs.
A Continuing Broker Role
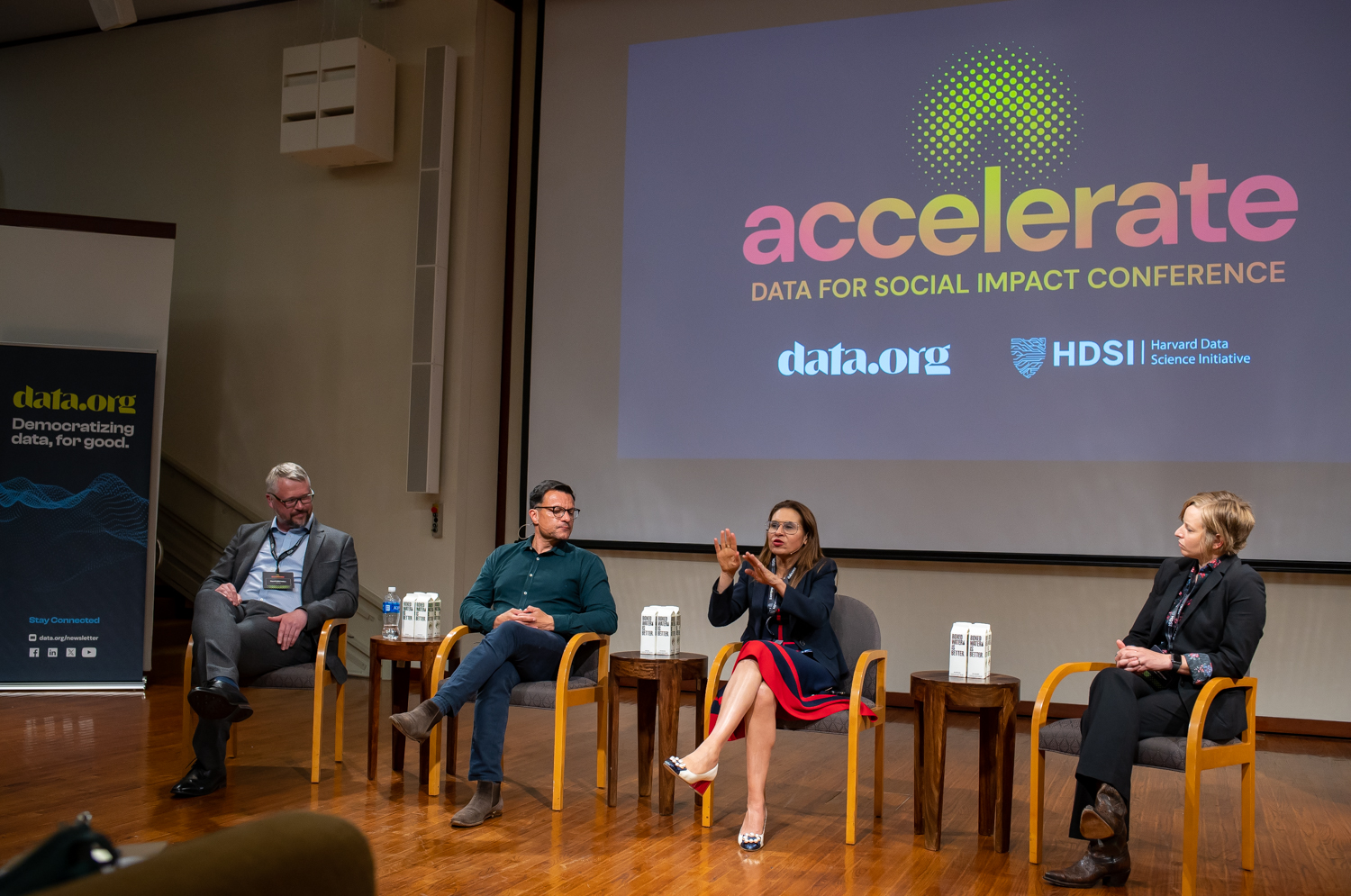
Finally, mapping this rapidly evolving landscape is an ongoing challenge. There’s a critical opportunity for neutral brokers, like data.org or other convening bodies, to maintain and update taxonomies of AI for Good. By doing so, they can help nonprofits find trusted partners, highlight responsible AI guidelines, and keep the momentum going for breakthroughs that genuinely change lives.
Since our first deep dive into Data for Good efforts, the field has grown tremendously – with actors moving from building to accelerating. More data, more models, more funding, and more guidance than ever before are flowing into mission-driven AI endeavors. At the same time, more clarity is needed for organizations seeking to navigate this maze, particularly when it comes to problem scoping, program design, long-term implementation, and reporting. We hope this updated view of the AI for Good landscape provides a helpful roadmap for innovators, funders, and practitioners to find each other, collaborate, and continue driving real, transformative impact.
References
AI For Good Impact Report | Division for Inclusive Social Development (DISD). social.desa.un.org/sdn/ai-for-good-impact-report.
“AI for Good Lab – Microsoft Research.” Microsoft Research, 4 Feb. 2025, www.microsoft.com/en-us/research/group/ai-for-good-research-lab.
AscendixTech. “How Many AI Companies Are There in the World?” AscendixTech, 15 Jan. 2025, https://ascendixtech.com/how-many-ai-companies-are-there/.
data.org. “Charting the Data for Good Landscape – an Update.” data.org, 25 May 2022, data.org/news/charting-the-data-for-good-landscape-an-update.
Edge Delta. “The Future is Now: AI Startup Statistics in 2024.” Edge Delta, 2024, https://edgedelta.com/company/blog/ai-startup-statistics.
Esser, Kat, et al. The Food Value Chain X Cloud Technology: A Landscape Analysis – North America. 2024, pages.awscloud.com/rs/112-TZM-766/images/AWS_Deloitte_Food_Value_Chain_X_Cloud_Technology.pdf.
Generosity AI Working Group. AI Readiness Survey Report 2024. GivingTuesday, 2024, https://ai.givingtuesday.org/ai-readiness-report-2024/#organizational-capacity-and-ai-readiness.
Hall, Brian. “Real-world Gen AI Use Cases From the World’s Leading Organizations.” Google Cloud Blog, 19 Dec. 2024, cloud.google.com/transform/101-real-world-generative-ai-use-cases-from-industry-leaders.
Hinde, Gillian, et al. AI For Impact: Strengthening AI Ecosystems for Social Innovation. report, 2024.
“Meet Five Organisations Using AI for Social Good.” EU About Amazon, 19 Apr. 2024, www.aboutamazon.eu/news/aws/meet-five-organisations-using-ai-for-social-good.
Panel, Expert. “How Tech Companies Can Grow Their Social Influence and Impact.” Forbes, 23 May 2024, www.forbes.com/councils/forbestechcouncil/2024/05/22/how-tech-companies-can-grow-their-social-influence-and-impact.
The AI for Social Good landscape is dynamic and rapidly evolving. The information presented in the report represents a snapshot in time and may not reflect the full scope or future direction of ongoing efforts in this space.
About the Authors
Perry Hewitt is the Chief Marketing and Product Officer of data.org where she oversees the marketing and communications functions, as well as digital product development.
Read moreJake Porway loves seeing the good values in bad data. As a frustrated corporate data scientist, Porway co-founded DataKind, a nonprofit that harnesses the power of Data Science and AI in the service of humanity by providing pro bono data science services to mission-driven organizations.
Read more