The CAN Evaluation was led by Outlier Research & Evaluation at the University of Chicago. The evaluation took place in two phases. First, Outlier worked with University of Chicago leadership to articulate an overall Theory of Action for the CAN Network. Then, the evaluation focused on outcomes for the UChicago Data Science for Social Impact (DSSI) Summer Program.
Theory of Action
The image below illustrates the intention behind CAN and how it was organized. First, with funding from data.org, the committed leaders of the CAN network partners convened. They started by creating norms for their collaboration and then moved their data science for social impact work forward with coordinated and ongoing communication. The result of this work has been the programs described in this Playbook. They continue their work to impact both their students and their institutions in order to move toward a long-term vision of increased numbers and diversity in data science and increased application of data science for social impact.
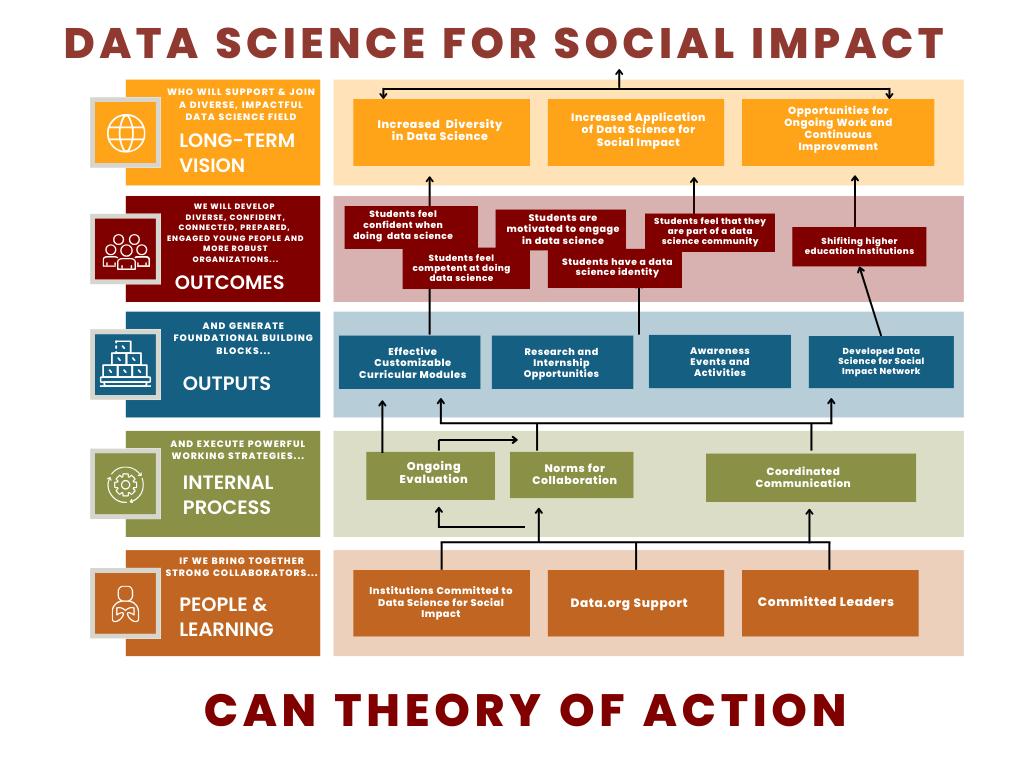
Data Science for Social Impact (DSSI) summer program evaluation
At the outset, Outlier worked with DSSI program leaders to identify measurable target outcomes. Next, they developed a pre-questionnaire to measure participants’ baseline for the target outcomes. All participants completed the questionnaire on the first day of the program. To measure the change in target outcomes, the participants completed a post-questionnaire, which included identical items found on the pre-questionnaire. The post-questionnaire also contained questions that measured students’ experience in the program and solicited program improvement feedback.
Outcomes measured included: a) content familiarity; b) self-efficacy; c) prediction for future success; d) perception of self; e) interest/motivation to complete challenge data science; f) interest in data science in general; g) self-efficacy: project management; h) self-efficacy: client work; and i) sense of belonging.
Other outcomes measured focused on what the DSSI participants anticipated doing next. They were asked about a) the likelihood of pursuing additional data science education; b) the likelihood of pursuing a career related to data science; and c) the sector of data science career (if pursuing a data science career).
Finally, if participants anticipated pursuing future education in data science or a data science career, they were asked about support for taking these next steps that they felt they did and did not have. These included: a) sufficient knowledge about the education they needed; b) sufficient financial support/knowledge about how to obtain financial support for education; c) sufficient personal/familial support; d) sufficient knowledge about career pathways; e) sufficient knowledge and skills in the content; f) sufficient financial support for basic needs.
Questionnaire findings were complemented by data from participant interviews. Ten different participants (out of 24) were interviewed with six interviewed at the program midpoint and four interviewed during the final week of the program.
The evaluators produced two deliverables, which were shared with the DSSI program leaders and instructors. The first was shared a few weeks into the program and contained descriptive data from the pre-questionnaire. The second was shared after the conclusion of the program and presented quantitative and qualitative findings from the questionnaires and interviews as well as recommendations for program improvement.